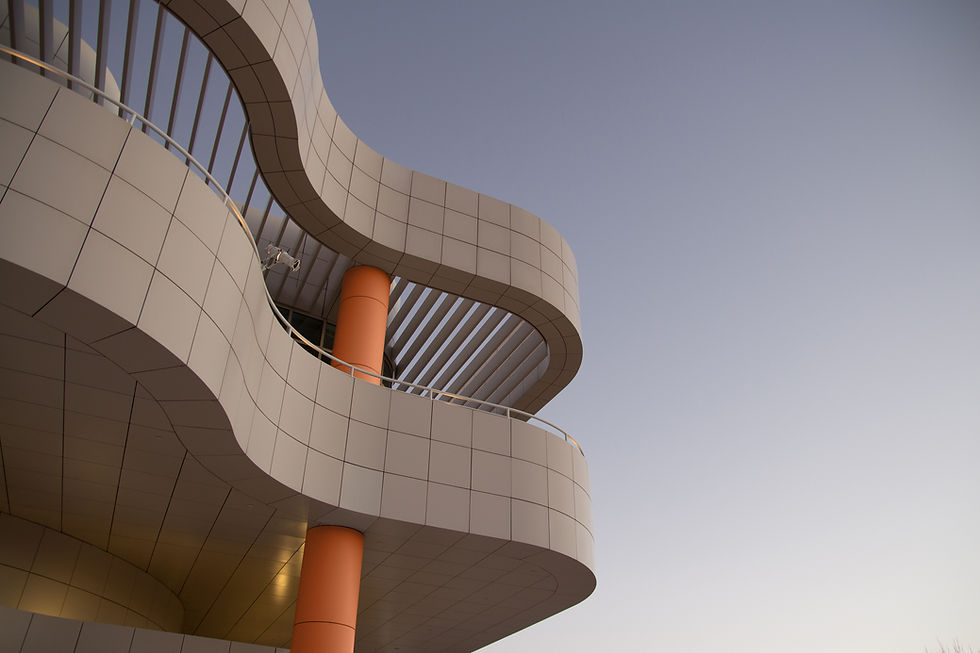
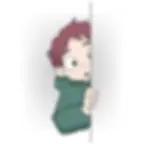
Amidst the magical wizardry that customers and consumers hear about, read about, and actually use every day, organizations are still falling alarmingly short of customer experience expectations.
Agentic AI now employs advanced reasoning abilities to enhance the complete customer experience. It is capable of comprehending and addressing customer service inquiries, forecasting and influencing purchasing behavior with hyper personalized lookalike modeling, as well as making decisions and automating tasks across marketing, fulfillment, and service operations. Additionally, Agentic AI continuously improves itself through in-depth pattern analysis, language and voice recognition, and intentional learning.
Agentic AI follows a cognitive process to do its job at a speed, efficiency, and scale that is outside the realm of human capability. An AI agent collects and processes data from a vast data sets and a range of data sources, such as transaction history, customer service interactions, shopping modalities, etc., in real-time. Using deep learning, the agent identifies patterns from the data and makes decisions, and may also use a large language model to figure out tasks, generate solutions, and orchestrate other models, such as recommendation engines or generative content systems. Next, the agent executes the decisions into action, by integrating with other tools and applications via APIs to perform tasks, within pre-set rules and guidelines. The AI agent learns and improves continuously based on data from past interactions, and also updates its knowledge base/model to enhance future performance.
In order for Agentic AI to enable an organization to elevate the customer experience and match customer expectations, a myriad of factors must be thrown up on the white board in a room full of experts, inclusive of internal talent and trusted outside partners. These include:
Data: AI models need to be trained on high-quality data, which is consistent, complete, accurate, and free of bias, to produce matching algorithmic outcomes. Also, data sourcing, consistency, taxonomy, privacy and regulatory adherences must be assessed and planned in detail.
Technology: the technology platforms driving the data flow, the enterprise operations and the customer experiences need to enable and support the Agentic AI capabilities and use cases. Many if not most of the enterprises I have worked with have inevitable gaps in their current platform systems and capabilities. The speedy evolution of MACH architecture (Microservices, API-first, Cloud-native, and Headless) allows unprecedented flexibility so that enterprises can deploy microservices that drive curated digital journeys in a matter of weeks, in most cases regardless of their existing systems.
Organizational readiness: the most challenging factor in successful Agentic AI deployment can often be organizational readiness. Talent, leadership, organizational structure and change management are key factors in the ability of Agentic AI to add value and feasibility to critical enterprise use cases.
To overcome the complexity and challenges of realizing tangible value from AI, enterprises should always deploy expert resources and partners to ensure their AI investments are strategized, planned, assessed and implemented effectively and transparently, allowing for unobstructed oversight and thorough governance by a well structured team.